Table Of Content
- Contrasts, main effects and interactions
- How to Read CSV File from String into Pandas DataFrame
- Durbin-Watson Table
- Interaction between Distraction and Reward
- 8.3 Conduct the ANOVA
- 3.5. Identifying main effects and interactions¶
- 5: Practice with a 2x2 Factorial Design- Attention
- How to Count Unique Values in Column in R

To test all of these conditions simultaneously, a two-by-two factorial design is required. Rather than test many potential influences one experiment at a time, a factorial design allows the simultaneous examination of several variables within one experiment. Such design requires fewer participants, and reveals whether the various causes interact in a special way to affect the outcome. As these researchers expected, participants who were lower in SES tended to give away more of their points than participants who were higher in SES. This is consistent with the idea that being lower in SES causes people to be more generous.
Contrasts, main effects and interactions
Nonverbal communication deciphering by self-esteem and self-awareness. Contrary to the hypothesized pattern, notice that participants with high self-awareness and low self-esteem were more accurate at deciphering nonverbal expressions. However, when exposed to low self-awareness, participants were more accurate when they had high self-esteem. Provide them with informed consent, a brief description of the research, sense of the procedure, the potential risks and benefits of participating, and the right to withdrawal at any time. Answer the following questions to understand the variables and groups that we are working with.
Adding abiraterone to ADT/docetaxel may be new standard in de novo mCSPC - Urology Times
Adding abiraterone to ADT/docetaxel may be new standard in de novo mCSPC.
Posted: Mon, 20 Sep 2021 07:00:00 GMT [source]
How to Read CSV File from String into Pandas DataFrame
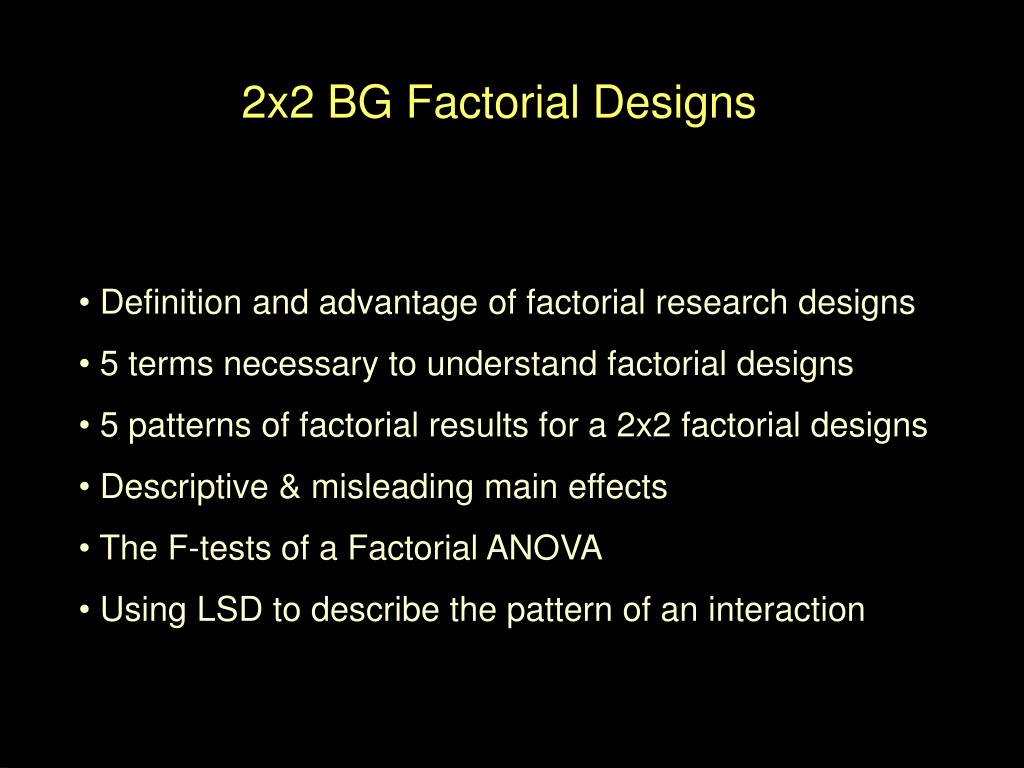
So, the main effect of wearing shoes is to add 1 inch to a person’s height. To briefly add to the confusion, or perhaps to illustrate why these two concepts can be confusing, we will look at the eight possible outcomes that could occur in a 2x2 factorial experiment. We have usually no knowledge that any one factor will exert its effects independently of all others that can be varied, or that its effects are particularly simply related to variations in these other factors. There are various traditions to denote the levels of each factor.
Durbin-Watson Table
From the example above, suppose you find that 20 year olds will suffer from seizures 10% of the time when given a 5 mg CureAll pill, while 20 year olds will suffer 25% of the time when given a 10 mg CureAll pill. When 40 year olds, however, are given a 5 mg pill or a 10 mg pill, 15% suffer from seizures at both of these dosages. There is an increasing chance of suffering from a seizure at higher doses for 20 year olds, but no difference in suffering from seizures for 40 year olds. Thus, there must be an interaction effect between the dosage of CureAll, and the age of the patient taking the drug.
Because experiments from the POD are time consuming, a half fraction design of 8 trial was used. The figure below contains the table of trials for the DOE. This main total effect value for each variable or variable combination will be some value that signifies the relationship between the output and the variable. For instance, if your value is positive, then there is a positive relationship between the variable and the output (i.e. as you increase the variable, the output increases as well).
This is done much like adding data into an Excel data sheet. In the columns to the right of the last factor, enter each response as seen in the figure below. The above table contains all the conditions required for a full factorial DOE. Minitab displays the standard order and randomized run order in columns C1 and C2, respectively.
Treatment combinations are denoted by ordered pairs or, more generally, ordered tuples. The parentheses are often dropped, as shown in the accompanying table. First, compute distraction effect for each subject when they were in the no-reward condition. Second, compute the distraction effect for each subject when they were in the reward condition. The overall means for for each subject, for the two reward conditions are shown to the right.
5: Practice with a 2x2 Factorial Design- Attention
Pareto charts for both wt% MeOH in biodiesel and number of theoretical stages are shown below. After all the trials were performed, the wt% methanol remaining in the biodiesel and number of theoretical stages achieved were calculated. The figure below contains the DOE table of trials including the two responses.
The interaction can test whether the effect of IV1 changes between the levels of IV2. When it does, researchers can infer that their second manipulation (IV2) causes change in their effect of interest. These changes are then documented and used to test underlying causal theories about the effects of interest. Let’s conduct a 2x2 repeated measures ANOVA on the data to evaluate whether the differences in the means are likely or unlikely to be due to chance.
We have already seen that factorial experiments can include manipulated independent variables or a combination of manipulated and non-manipulated independent variables. But factorial designs can also consist exclusively of non-manipulated independent variables, in which case they are no longer experiments but correlational studies. Consider a hypothetical study in which a researcher measures two variables. First, the researcher measures participants’ mood and self-esteem.
First, the researchers can say that standing up reduces the size of a person’s Stroop effect. Well, if the Stroop effect is an index of selective attention, then it could mean that standing up is one way to improve your ability to selectively focus and ignore distracting information. The actual size of the benefit is fairly small, so the real-world implications are not that clear.
For example, a researcher might choose to treat cell phone use as a within-subjects factor by testing the same participants both while using a cell phone and while not using a cell phone. But they might choose to treat time of day as a between-subjects factor by testing each participant either during the day or during the night (perhaps because this only requires them to come in for testing once). Thus each participant in this mixed design would be tested in two of the four conditions. This is a complex design with complex statistical analyses. In the remainder of this section, we will focus on between-subjects factorial designs only.
We see the red bar (tired) is 1 unit lower than the green bar (not tired). So, there is an effect of 1 unit of being tired in the 1 hour condition. Next, look at the effect of being tired only for the “5 hour” condition. We see the red bar (tired) is 3 units lower than the green bar (not tired). So, there is an effect of 3 units for being tired in the 5 hour condition. Clearly, the size of the effect for being tired depends on the levels of the time since last meal variable.
The additional complication is the fact that more than one trial/replication is required for accuracy, so this requires adding up each sub-effect (e.g adding up the three trials of a1b1). By adding up the coefficient effects with the sub-effects (multiply coefficient with sub-effect), a total factorial effect can be found. This value will determine if the factor has a significant effect on the outcome. For larger numbers, the factor can be considered extremely important and for smaller numbers, the factor can be considered less important. The sign of the number also has a direct correlation to the effect being positive or negative. Another independent variable, such as the administration of alcohol or placebo, allows for the investigation into how shock level and alcohol influence the startle response.
No comments:
Post a Comment